Dynamic Mortality Prediction in Critically Ill Children During Interhospital Transports Using Explainable AI
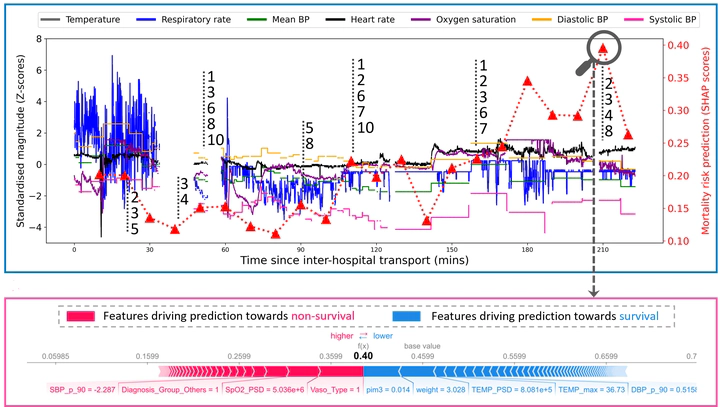
๐ AI-Driven Mortality Prediction for Interhospital Transport in Pediatric Intensive Care
We are thrilled to share our latest research on real-time mortality risk prediction in critically ill children during interhospital transport to paediatric intensive care units (PICUs). This study, published in npj Digital Medicine, introduces an explainable AI-powered model designed to enhance clinical decision-making in transport medicine.
๐ฌ Key Findings
- Real-time, dynamic risk prediction โ Continuously evaluates mortality risk based on vital signs, interventions, and transport conditions.
- Explainable AI with SHAP analysis โ Identifies key clinical contributors to risk estimation, enhancing trust and usability.
- Higher accuracy than traditional methods โ The PROMPT model outperforms PIM3, achieving AUROC of 0.83.
- Scalable deployment โ Designed for integration into ambulance monitoring systems and clinical decision-support tools.
๐ข Clinical Impact
This research provides clinicians with real-time, AI-driven insights to support early interventions, reduce preventable adverse events, and optimize transport outcomes. The PROMPT model paves the way for next-generation predictive analytics in pediatric intensive care transport.
๐ Read the full article here: Publication Link